What 70% of Data Science Learners Do Wrong ?
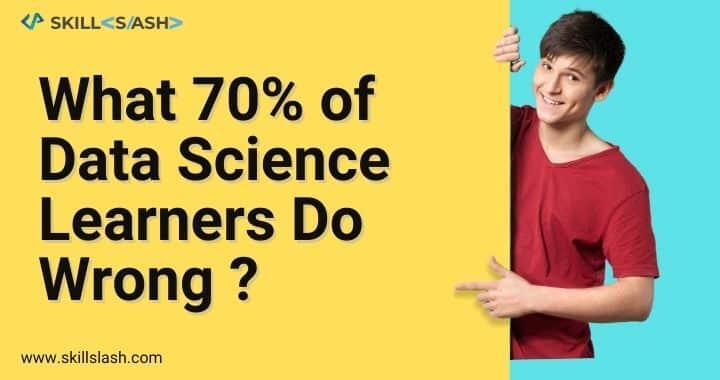
Data science has become an increasingly popular field in recent years, with a growing demand for professionals who can analyze and interpret large amounts of data to inform business decisions and solve complex problems. From predicting customer behavior to discovering new drugs and treatments, data science has the potential to transform a wide range of industries and drive innovation.
However, despite its potential and the many resources available for learning data science, many learners make common mistakes that can hinder their success and growth in the field. In this article, we will explore five common mistakes made by 70% of data science learners and offer suggestions for how to avoid them. By understanding and addressing these mistakes, data science learners can set themselves up for success and make the most of their learning journey.
Mistake #1: Underestimating the Importance of Math and Statistics
Math and statistics are fundamental to data science and are used in almost every aspect of the field, from data analysis and modeling to machine learning and data visualization. However, many data science learners underestimate the importance of math and statistics and do not prioritize improving their skills in these areas.
A lack of math and statistics knowledge can lead to several problems, including:
- Poor data analysis: If you don’t have a strong foundation in math and statistics, you may struggle to understand and analyze data effectively.
- Inaccurate modeling: If you don’t understand the statistical concepts and techniques used in modeling, you may develop models that are not accurate or reliable.
- Limited career opportunities: Many data science jobs require a strong foundation in math and statistics, so a lack of knowledge in these areas can limit your career opportunities.
So, what can you do to improve your math and statistics skills as a data science learner? Here are a few suggestions:
- Take online courses: There are many online courses and tutorials available that can help you improve your math and statistics skills.
- Practice with real-world data sets: Working with real-world data sets can be a great way to apply your math and statistics knowledge and improve your skills.
- Seek out resources and materials: There are many resources and materials, such as textbooks and online articles, that can help you learn more about math and statistics.
By taking steps to improve your math and statistics skills, you can set yourself up for success and growth as a data scientist.
Mistake #2: Not Paying Attention to Data Cleaning and Preparation
Data cleaning and preparation is a crucial step in the data science process, and it’s one that many learners overlook or underestimate. However, the quality of your data has a direct impact on the accuracy of your analysis and modeling, so it’s important to take the time to properly clean and prepare your data.
Some common pitfalls that learners may encounter when cleaning and preparing data to include:
- Not checking for missing values: If you don’t check for missing values in your data, you may end up with incomplete or inaccurate results.
- Not understanding the data’s structure and format: If you don’t understand the structure and format of your data, you may struggle to properly clean and prepare it for analysis.
- Not using appropriate libraries and tools: Using the wrong libraries or tools can make data cleaning and preparation more time-consuming and difficult.
So, what can you do to efficiently and effectively clean and prepare your data? Here are a few tips:
- Use appropriate libraries and tools: There are many libraries and tools available, such as pandas and OpenRefine, that can make data cleaning and preparation easier.
- Understand the structure and format of your data: Take the time to understand the structure and format of your data so that you can properly clean and prepare it.
- Check for missing values: Make sure to check for missing values and handle them appropriately.
By following these tips and taking the time to properly clean and prepare your data, you can ensure that your analysis and modeling are based on high-quality data.
Mistake #3: Not Knowing the Business Domain
As a data scientist, it’s important to have a deep understanding of the business domain in which you are working. This means understanding the industry, the company, and the specific problem or question that you are trying to solve. Without a solid understanding of the business domain, you may make incorrect conclusions or develop solutions that are not aligned with the needs of the business.
For example, if you are analyzing data for a healthcare company and you don’t have a deep understanding of the healthcare industry, you may draw incorrect conclusions or develop solutions that are not practical or feasible in a healthcare setting. Similarly, if you are analyzing data for a retail company and you don’t understand the company’s business model and customer base, you may develop solutions that are not aligned with the company’s goals.
So, how can you gain a deeper understanding of the business domain? Here are a few suggestions:
- Work on projects with domain experts: If you have the opportunity to work on a project with someone who has a deep understanding of the business domain, take advantage of it. This can be a great way to learn from someone who has real-world experience in the industry.
- Seek out resources and materials on specific industries: There are many resources available, such as industry reports and trade publications, that can help you learn about specific industries and businesses.
- Attend industry events and conferences: Attending industry events and conferences can be a great way to learn about the latest trends and developments in a particular business domain. You can also network with others in the industry and gain insights from their experiences.
By taking steps to gain a deeper understanding of the business domain, you can ensure that your data analysis and solutions are aligned with the needs of the business and are more likely to be successful.
Mistake #4: Not Practicing Enough
As a data scientist, hands-on experience and practice are crucial for becoming proficient in your craft. While online courses and academic programs can provide a strong foundation of knowledge, they can’t replicate the real-world experience and challenges that you’ll encounter on the job. Simply reading about data science concepts and techniques is not enough – you have to put them into practice to truly understand and master them.
However, many learners make the mistake of thinking that online courses alone are sufficient for gaining practical experience. This couldn’t be further from the truth. While online courses can certainly be a valuable resource, they should be supplemented with other forms of hands-on practice.
So, what can you do to get more practice as a data scientist? Here are a few suggestions:
- Participate in hackathons: Hackathons are events where you can work on real or simulated data science projects in a competitive environment. They provide an excellent opportunity to apply your skills and learn from others.
- Work on personal projects: Find a data set that interests you and try to solve a problem or answer a question using data science techniques. This can be a great way to get hands-on experience and try out new techniques.
- Collaborate with others: Working with others, whether in a team or as part of an online community, can be a fantastic way to learn and get feedback on your work. You can also learn from the experiences and approaches of others.
Remember, becoming a proficient data scientist requires more than just learning from online courses. Make sure to supplement your education with hands-on practice and experience to truly master the field.
Mistake #5: Not Having a Growth Mindset
As a data science learner, having a growth mindset can make a huge difference in your success and career development. A growth mindset is a belief that your abilities and intelligence can be developed and improved through effort, learning, and practice. On the other hand, a fixed mindset is the belief that your abilities are fixed and cannot be changed.
Having a fixed mindset can hold you back as a data scientist in several ways. For example, if you believe that you are not naturally good at math and statistics, you may be less likely to put in the effort to improve your skills. Similarly, if you are afraid to ask questions or try new things, you may miss out on valuable learning opportunities.
On the other hand, having a growth mindset can help you embrace challenges and seek out feedback to improve your skills. It can also help you stay motivated and resilient in the face of setbacks and failures.
So, how can you cultivate a growth mindset as a data science learner?
Here are a few tips:
- Embrace challenges: Don’t shy away from difficult tasks and problems – embrace them as opportunities to learn and grow.
- Seek out feedback: Ask for feedback from others on your work and use it to identify areas for improvement.
- Learn from failures: Don’t see failures as a sign of your limitations, but rather as opportunities to learn and do better next time.
By cultivating a growth mindset, you can set yourself up for success and continuous learning as a data scientist. Don’t let a fixed mindset hold you back – embrace challenges and seek out opportunities to grow and improve.
Conclusion
A significant portion of data science learners make several common mistakes in their journey toward becoming proficient in the field. These mistakes include focusing too heavily on theory and not enough on practical application, failing to build a strong foundation in mathematics and statistics, and not seeking out diverse learning opportunities. It is important for aspiring data scientists to be mindful of these pitfalls and actively work to avoid them to truly succeed in this rapidly-growing and competitive field. By staying focused, staying curious, and staying determined, anyone can become a successful data scientist with the right mindset and approach.
The Advanced Data Science and AI program by Skillslash is the ultimate opportunity for aspiring professionals to take their careers to the next level. Not only does the program cover all the key concepts and tools needed to succeed in today’s data-driven world, but it also provides students with valuable real-world experience through internships with top AI startups. These internships not only give students the chance to apply their knowledge in a professional setting but also provide them with project certification to boost their resumes and increase their employability. In addition, Skillslash offers unlimited job referrals to help graduates of the program get placed at top companies in the field. With its comprehensive curriculum, expert instruction, and real-world experience, the program is the ultimate investment in your future.
Moreover, Skillslash also has in store, exclusive courses like Data Science Course In Kolkata, Full Stack Developer Course and Web Development Course In Hyderabad to ensure aspirants of each domain have a great learning journey and a secure future in these fields. To find out how you can make a career in the IT and tech field with Skillslash, contact the student support team to know more about the course and institute.