Unraveling the Genetic Mysteries: The Role of Machine Learning in Genomics
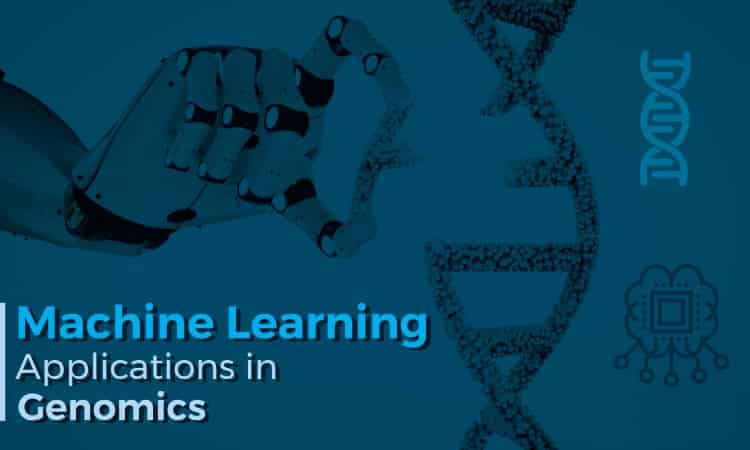
In the ever-evolving landscape of science and technology, the fusion of Machine Learning and genomics has emerged as a groundbreaking alliance, poised to revolutionize our understanding of genetics and genomics. As we venture deeper into the intricate realms of our DNA, Machine Learning solutions have proven to be indispensable tools for researchers, offering unprecedented insights into the genetic mysteries that have long perplexed us. This article delves into the fascinating world of Machine Learning in genomics, exploring the synergy between genetics and Machine Learning and the transformative potential it holds.
Machine Learning in Genomics: A Powerful Partnership
Genomics, the study of an organism’s complete set of DNA, has unlocked a treasure trove of data over the years. With the advent of high-throughput sequencing technologies, we now possess an unimaginable amount of genetic information. However, the challenge lies in interpreting this vast amount of data, and this is where Machine Learning steps in.
Machine Learning in genomics involves the application of algorithms and statistical models to analyze and make predictions based on genomic data. It has the power to sift through mountains of genetic information to uncover patterns, associations, and insights that would be virtually impossible for humans to discern alone. With Machine Learning for genomics, we can harness the full potential of genetic data to advance our understanding of diseases, evolution, and the fundamental building blocks of life itself.
Machine Learning and Genomics: Transforming Healthcare
One of the most promising applications of Machine Learning in genomics is in the field of healthcare. Machine learning solutions are revolutionizing the way we diagnose and treat genetic diseases. By analyzing the genetic makeup of individuals, Machine Learning algorithms can predict disease risk, guide treatment choices, and even personalize medical interventions.
For example, in cancer genomics, Machine Learning can identify genetic mutations that contribute to the development and progression of various cancers. This knowledge enables healthcare providers to tailor treatment plans to a patient’s specific genetic profile, increasing the chances of successful outcomes. Additionally, Machine Learning is used in drug discovery, where it accelerates the identification of potential therapeutic targets and the design of novel drugs.
Genetics and Machine Learning: An Interdisciplinary Marvel
The fusion of genetics and Machine Learning has created a new interdisciplinary frontier. Geneticists and computer scientists are collaborating more closely than ever to extract meaningful insights from genomic data. This collaborative effort has led to the development of innovative Machine Learning algorithms designed specifically for genomics, further fueling discoveries in the field.
One such example is the use of deep learning, a subset of Machine Learning, in genomics. Deep learning algorithms, such as convolutional neural networks (CNNs) and recurrent neural networks (RNNs), have demonstrated remarkable success in tasks like DNA sequence analysis and prediction of gene expression. These models can automatically learn and extract complex patterns from genomic data, leading to improved accuracy and efficiency in various genomics applications.
Machine Learning Solutions for Genetic Mysteries
Machine learning solutions have proven invaluable in solving some of the most intriguing genetic mysteries. Consider the challenge of understanding the genetic basis of complex traits and diseases. Machine learning models can analyze vast datasets, including genome-wide association studies (GWAS), to pinpoint specific genetic variations associated with conditions like diabetes, heart disease, and mental illnesses. This knowledge not only deepens our comprehension of these conditions but also opens doors for the development of novel therapies and interventions.
Furthermore, Machine Learning is aiding in the reconstruction of ancient DNA and the study of human evolution. By analyzing the genetic information extracted from ancient specimens, researchers can trace the migration patterns of our ancestors, shedding light on the tapestry of human history. Machine learning algorithms play a pivotal role in processing and interpreting this wealth of genetic data.
Conclusion: The Future of Genomics
As we stand on the precipice of the future, the integration of Machine Learning in genomics promises to unravel the most enigmatic genetic mysteries. The synergy between genetics and Machine Learning is reshaping healthcare, advancing our understanding of the human genome, and catalyzing groundbreaking discoveries. With Machine Learning solutions at our disposal, the boundaries of genetic exploration are pushed ever further, offering hope and illumination in the quest to decipher the intricate language of our DNA.
In conclusion, the role of Machine Learning in genomics is a testament to human ingenuity and the power of interdisciplinary collaboration. It is a remarkable journey that has only just begun, holding the potential to transform our understanding of genetics and unlock a future where personalized medicine and tailored interventions are the norm. Genetics and Machine Learning are no longer distinct entities but partners in the relentless pursuit of genetic knowledge, bringing us closer to the ultimate unraveling of the intricate genetic mysteries that have captivated scientists for generations.