Unleashing the Power of Analytics in Storage Management
In the realm of IT infrastructure, data storage is the unsung hero whose significance often goes unnoticed until a disk crashes or a system reaches ...
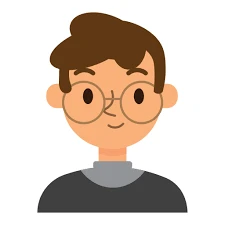
In the realm of IT infrastructure, data storage is the unsung hero whose significance often goes unnoticed until a disk crashes or a system reaches ...