Standard Operating Guide for Nonclinical Data Exchange
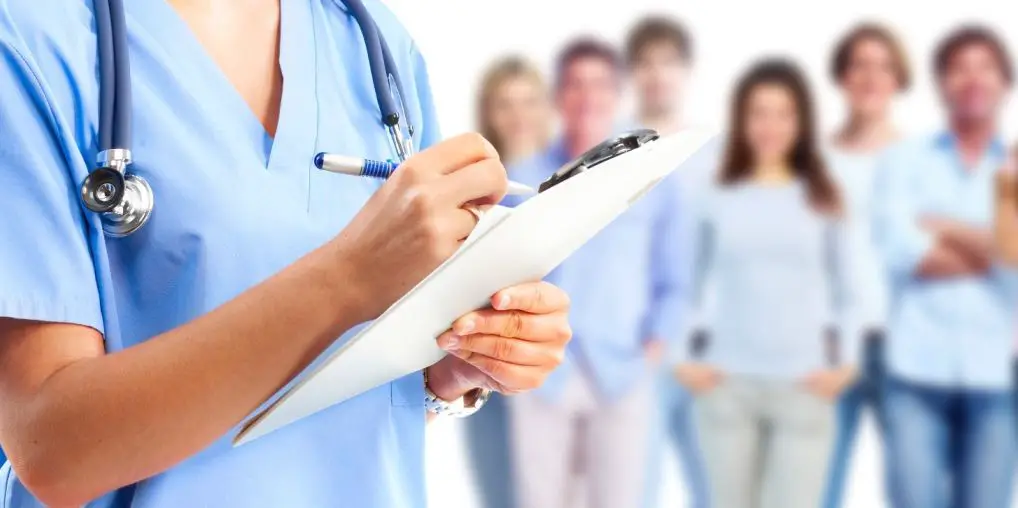
I. Overview
The Nonclinical Data Exchange Standard is a guideline for standardizing and unifying the exchange of nonclinical experimental data, aiming to improve data sharing and interoperability. This operation guide will guide users to perform operations on standardized data elements, data storage formats, data transmission protocols, data security, data integrity, data readability, data scalability and data compliance.
2. Standardized data elements
- Define standardized data elements: In data exchange, standardized data elements need to be defined, including experimental types, experimental animals, administration methods, observation indicators, etc.
- Unified naming and coding: Develop unified naming and coding rules for each data element to ensure the consistency of data elements in data exchange.
- Develop a data element dictionary: Establish a data element dictionary to clarify the meaning, scope and usage of each data element.
3. Data storage format
- Choose a common data storage format: It is recommended to use a common data storage format that complies with international standards, such as XML, CSV or JSON.
- Define a structured data model: Based on the characteristics of the experimental data, design a structured data model to facilitate data organization and storage.
- Ensure data readability and parsability: Use clear data structures and tags to ensure data readability and parsability.
4. Data transmission protocol
- Choose a common data transmission protocol: It is recommended to use common protocols such as HTTP or FTP for data transmission.
- Define API interface: According to business needs, define API interface to realize automatic transmission and acquisition of data.
- Ensure data transmission efficiency and security: optimize transmission methods, improve data transmission efficiency, and ensure security during data transmission.
5. Data Security
- Ensure network security: Ensure the network security of data by using encryption technology, access control, firewalls and other measures.
- Data privacy protection: Develop strict data usage regulations to ensure the privacy and confidentiality of experimental data.
- Data backup and recovery: Back up data regularly and develop corresponding recovery strategies to prevent data loss.
6. Data integrity
- Design a complete data collection process: To ensure the integrity of the data, the entire process from experimental design to data collection, sorting and analysis needs to be strictly controlled.
- Data verification and verification: Verify and verify the data to ensure the accuracy and completeness of the data.
- Data deduplication and conflict resolution: Deduplication and resolution of possible duplicate data or conflicting data.
7. Data readability
- Use clear data formats and tags: To ensure the readability of data, you need to adopt clear data formats and tags to make it easy for other users to understand and use the data.
- Provide necessary data annotations and documentation: Provide necessary annotations and documentation for the data so that other users can understand the meaning and context of the data.
- Data visualization: Improve the readability and understandability of data through data visualization technology.
8. Data scalability
- Design scalable data structures: To ensure data scalability, you need to design scalable data structures to adapt to future data growth and changes.
- Adopt open data formats and protocols: Adopt open data formats and protocols to adapt to future changes in technology and needs.
- Consider future business needs and development trends: When formulating standards, future business needs and development trends need to be considered in order to adapt to future changes.
9. Data Compliance
- Comply with relevant laws, regulations and ethical norms: In non-clinical data exchange, it is necessary to comply with relevant laws, regulations and ethical norms to ensure data compliance.
- Develop internal policies and regulations: Develop internal policies and regulations to standardize the collection, use and processing of data and ensure data compliance.