Differences Between Big Data and Data Analytics
Data analytics and Big Data are often used interchangeably, but there are important distinctions to make when discussing the two topics. Understanding
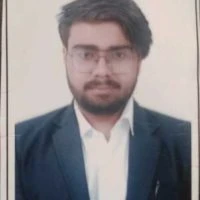
Data analytics and Big Data are often used interchangeably, but there are important distinctions to make when discussing the two topics. Understanding