Developing a Comprehensive Path to AI Maturity
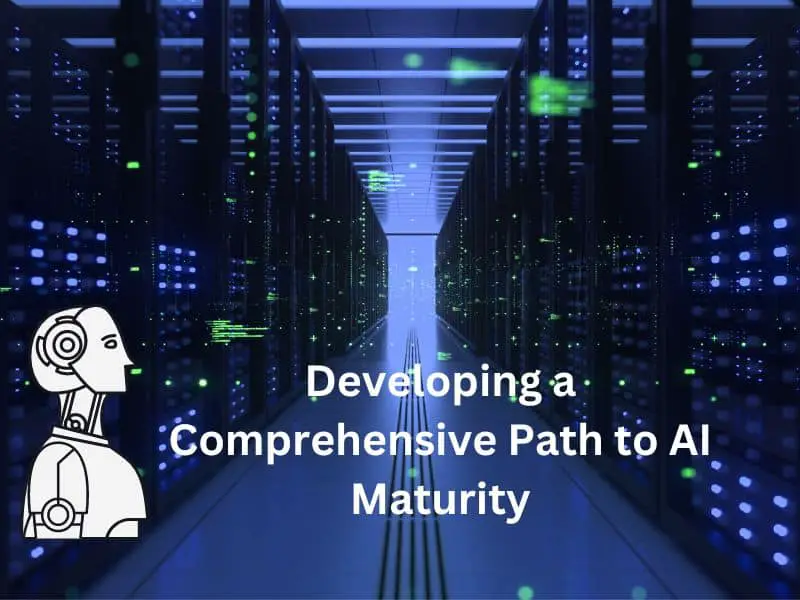
Introduction to Artificial Intelligence
If you’re wondering what Artificial Intelligence (AI) is and what it can do for your organization, then this section is for you. AI has become increasingly popular in recent years, as organizations are seeking out ways to gain a competitive edge. We’ll explore the various definitions of AI as well as its capabilities and technology stack. We’ll also look at an AI maturity framework and the benefits of mature AI implementations. Finally, we’ll review the best practices for adopting an agile approach to pilot projects, engaging key stakeholders in the process, and proactively managing risk.
What is Artificial Intelligence?
At its core, AI can be defined as the ability of machines or computer systems to perform tasks that would normally require human intelligence. This could include things like pattern recognition and machine learning algorithms that allow computers to improve their accuracy over time with more data. AI can also be used to automate processes, allowing humans to spend less time on mundane tasks while focusing on more complex tasks instead.
AI Capabilities & Technology Stack
The capabilities of AI are only limited by our imagination when it comes to potential applications. These could range from using natural language processing (NLP) to power virtual assistants, facial recognition technology for security purposes, object detection with drones or robots, or even automated decision-making based on data from previous outcomes. Check out : Data Analytics Courses in India
Exploring the Potential of AI
Exploring the Potential of AI has become a major focus for businesses and organizations all around the world. With its ability to automate processes and improve efficiency, it’s clear why so many are investing in this technology. But what exactly is the path to achieving AI maturity?
First, it’s important to understand the key benefits that AI has to offer. These include greater efficiency and cost savings in numerous aspects of operations. By leveraging AI technologies, organizations can better manage their resources and use them more effectively. Additionally, AI can provide an improved understanding of customer behavior and needs, making it easier for businesses to personalize their services accordingly. Finally, companies can leverage AI to automate mundane tasks, allowing employees time for more creative endeavors or increased focus on providing customers with exceptional experiences.
To realize these benefits though, organizations must be able to develop a plan for integrating AI into their operations and successfully implement it on time. This requires careful consideration of both technological feasibility as well as ethical implications of using this technology. Furthermore, organizations must make sure they have the right data resources available before they move forward with any automation initiatives – without clean data, projects often fail before they even get off the ground.
Then come to the skills required of those involved in managing AI projects. Companies must ensure they have individuals with excellent technical knowledge as well as business acumen so that their efforts are productive toward achieving goals that drive real ROI (return on investment). And this expertise isn’t limited just to tech-savvy folks; people from all departments should have some degree of knowledge about how AI works and how it may be applied in various contexts within their organization.
Key Technologies in AI Development
As we explore the path to AI maturity, it is important to understand the key differentiators of each technology for us to make informed decisions about which ones to deploy for our specific use cases.
Machine learning is a form of AI development that enables computers to automatically identify patterns and make predictions from data without being explicitly programmed. With machine learning algorithms, data scientists can rapidly develop solutions for difficult problems such as image recognition and pattern analysis. Natural language processing (NLP) is another foundational technology used in AI development. NLP uses techniques such as sentiment analysis and text classification to look for relationships between words in an unstructured corpus of text. This technology helps us rapidly understand human language and better connect with customers on their terms. Check out : Data Science Colleges in Mumbai
Deep learning algorithms are a type of machine learning algorithm that uses layered architectures, or “neural networks”, to represent complex situations. Unlike traditional machine learning, deep learning algorithms can determine correlations between inputs without having explicit labels associated with them. This makes them useful in situations where large amounts of unlabeled data need to be analyzed in order to identify patterns and classify images or objects accurately.
Adopting a Holistic, Cross-Industry Promotion of AI
Today, artificial intelligence (AI) is becoming increasingly recognized as an integral technology that is enabling the transformation of many industries. As such, understanding the path to AI maturity and cross-industry promotion is essential for businesses that are looking to take advantage of AI’s potential. In this blog section, we will explore how a holistic, cross-industry promotion of AI can be achieved by looking at certain essential components.
The first component in adopting a holistic approach to promoting AI is to recognize the AI maturity level of both individual organizations and entire industries. Each organization needs to take stock of its current capabilities in terms of infrastructure, talent availability, and data readiness before embarking on any adoption initiatives. Furthermore, collaboration with other industries must also be taken into account since many technologies today transcend boundaries.
The second important component for implementing a successful cross-industry promotion of AI is multi-stakeholder involvement from both the public and private sectors. Government bodies play an important role in providing policy guidance, setting rules and regulations, and promoting the use of existing standards and protocols when it comes down to harnessing the power of AI. Private sector entities provide ideas for technology development as well as promising use cases for further innovation. This type of collaboration allows both public and private stakeholders to benefit one another as well as society at large.
Building an Effective Analytics Platform for Enhanced Performance
In today’s increasingly data-driven economy, business success depends on having the right tools for gathering, analyzing, and utilizing data. A well-crafted analytics platform is at the heart of effective decision-making and performance optimization. To build an effective analytics platform for enhanced performance, there are several key components that you should consider.
First, is the process of data collection. You need to determine the necessary sources of data and have a plan in place to obtain them. This can be done through manual entry and automation depending on the types of data being collected. Once collected, you’ll need to analyze the data to uncover insights and make improvements. Here AI capabilities can help you develop better models for understanding relationships between variables and creating predictive insights based on large datasets. Check out : Data Science Training in Bangalore
Next are performance metrics – what measurements do you need to track from day to day? You’ll want to keep tabs on things like customer engagement rates, website traffic, lead conversions, employee productivity, etc., as these will provide invaluable feedback on how your business is performing compared to predetermined goals. Model governance is essential here as well because, without strong controls in place, these performance measurements can become a source of bias or miscommunication which can negatively impact outcomes.
Finally, automation and machine learning come into play as they offer scalability gains that traditional methods simply cannot match. Automation can take over mundane tasks such as data entry while machine learning algorithms can be applied to uncover real-time analytical insights that help teams make more informed decisions with accuracy. These technologies can save hours of time while also increasing consistency in measuring trends over time with greater accuracy than before possible.