Data Analytics Course: What are the disadvantages of Data Analytics? | Intellipaat
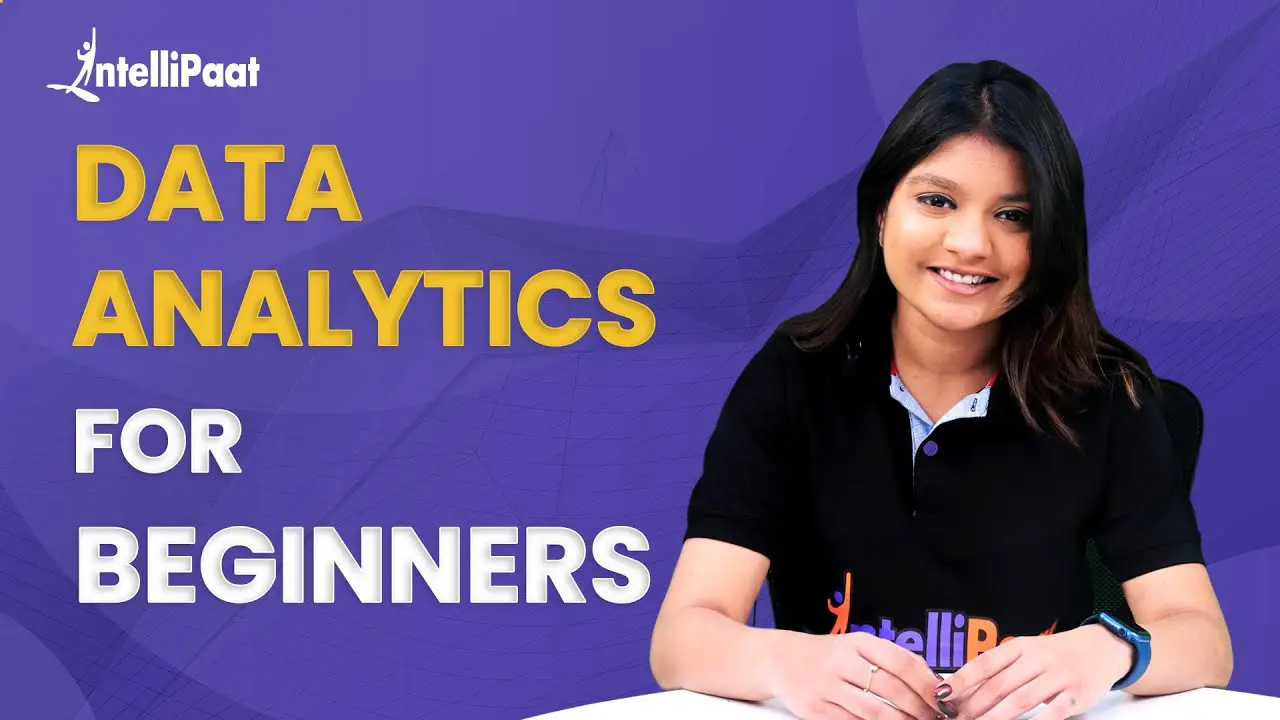
While data analytics has numerous advantages, there are also several disadvantages to consider, including:
-
Limited by data quality: The quality of the analysis is limited by the quality of the data. If the data is incomplete, inaccurate, or biased, the results of the analysis will be similarly affected.
-
Complexity: Data analytics can be complex and requires specialized knowledge and skills. Analyzing large datasets and building complex models can be time-consuming and require advanced technical expertise.
-
Limited by available data: The analysis is limited by the data that is available. If the data does not contain the necessary variables or is not representative of the population of interest, the results may be misleading.
If you want to learn more about Data Validation check out our Data Analytics Course video on YouTube. Our course covers everything you need to know about these types of analytics and how to effectively use them to drive informed decision-making.
-
Overreliance on algorithms: Data analytics often relies heavily on algorithms, which may not always be appropriate or accurate for the specific data or problem being analyzed. Additionally, the use of algorithms may limit the ability to interpret and explain the results of the analysis.
-
Privacy concerns: Data analytics may involve the collection and use of personal data, which raises concerns about privacy and data security. There is also the risk that personal data could be misused or used to discriminate against certain groups.
-
Ethical considerations: Data analytics raises ethical considerations, such as the potential for bias, discrimination, and unintended consequences. It is important to consider the ethical implications of the analysis and ensure that it is conducted in a responsible and transparent manner.
It is important to be aware of these potential disadvantages and to address them appropriately in the data analytics process. This includes ensuring data quality, using appropriate methods and algorithms, being transparent about the analysis and its limitations, and considering the ethical implications of the analysis.