Achieving Excellence in a Data Science Community Tips
Achieving excellence in a data science community is something that requires proper roles and strategies. Data science applies to nearly every aspect of modern life, making it an invaluable resource for any organization. As technology continues to evolve, the need for professionals who can help us develop data-driven solutions only increases.
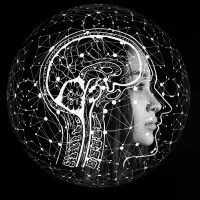