Best Practices for Effective Data Cleansing: A Guide for Businesses
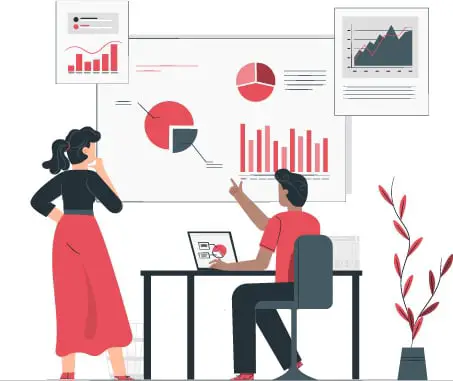
Poor quality data cannot be used for analysis or decision-making, as it won’t yield any authentic or reliable results. Instead, it leads to process inefficiencies, hampered productivity, impeded sales and marketing efforts, missing opportunities, and financial losses. Therefore, database cleanup services become necessary for organizations, whether B2B or B2C.
Data cleansing, also known as data cleaning or data scrubbing is a critical process for businesses to ensure the quality, accuracy, and consistency of their data. By removing inaccuracies, inconsistencies, and outdated information, businesses can enhance customer experiences, improve decision-making, and increase operational efficiency. Here are some best practices for effective data cleansing:
- Define Data Quality Standards
Establish data quality standards and create data quality key performance indicators (KPIs) and metrics specific to your business needs. Identify the key attributes that should be relevant, accurate, and complete, and establish guidelines for data entry and validation.
- Perform a Comprehensive Audit
Conduct an initial data audit of your existing database or data repository to identify inconsistencies, inaccuracies, and duplicates. This step helps you to understand the scope and scale of data quality issues so that you can devise your cleansing strategy accordingly.
- Define Data Cleansing Rules
Develop a set of data cleansing rules or algorithms to identify and correct errors automatically. These rules can include formatting standards, validation checks, and data enrichment techniques to better the overall quality of the data.
- Employ Data Profiling Techniques
Data profiling involves analyzing data to understand its content, structure, and relationships. It helps in identifying anomalies, missing values, and outliers. Profiling can either be done using automated tools or custom scripts to gain insights into the quality of the data.
- Remove Duplicates and Inconsistencies
Duplicate records and inconsistent entries can lead to inaccurate analysis and decision-making. Use data deduplication techniques to identify and remove duplicate records. Use fuzzy matching algorithms to identify similar records that may have variations in spelling or formatting and ensure that all such entries are removed or fixed.
- Validate and Standardize Data
Implement data validation checks to ensure its integrity and accuracy are intact. Validate data against predefined rules or reference data sets. In addition, standardize data formats such as addresses, phone numbers, and names to ensure consistency.
- Opt for Data Enrichment
As the name suggests, data enrichment involves enhancing your existing data with additional information gathered from external sources. This process can include appending geolocation data, demographic data, or social media data to enrich customer profiles and improve the quality of data.
- Regularly Update And Maintain Data
Maintaining high data quality is an ongoing effort. Establish processes to regularly update and maintain the quality of your data. This includes monitoring data sources, conducting regular data audits, and implementing data governance practices to ensure data remains accurate and up to date at all times.
- Provide User Training and Guidelines
It is important to educate your staff about data quality practices as well as provide them with guidelines for healthy data entry and management practices. Ensure that employees understand the importance of good quality data for organizations and their role in maintaining it.
- Use Technology and Automation
Leverage the most-modern data cleansing tools and technologies to automate the process as much as possible. There are various software solutions available that can help you identify and correct data quality issues efficiently. Other than this, you can outsource data cleansing services to get professional help. They deliver high-quality, accurate, and up-to-date databases within the stipulated time and budget.
- Implement a Data Governance Framework
Establishing a data governance framework helps in defining roles, responsibilities, and processes for effective data management within an organization. This also ensures accountability and ownership of data quality throughout the company.
- Monitor and Measure Data Quality
To keep a check on the quality of your data, it is advised to continuously monitor and measure the DQ using predefined metrics and key performance indicators (KPIs). Regularly evaluate the effectiveness of your data cleansing efforts and make adjustments as needed to prevent the future costs of data validation and verification.
Bottom Line
Overall, access to high-quality data accelerates the decision-making process, giving you an edge over your competitor. Effective data cleansing is an iterative process that requires continuous improvement and maintenance. Committing to a regular data cleansing practice will produce good data, which is the key to cutting through the competition and carving a unique niche in the industry. By following the above-mentioned best practices, organizations can ensure their data remains accurate, reliable, and valuable for making informed decisions.
While choosing the right data cleansing services company, ask for their client testimonials, check the quality of past projects delivered, assess whether they have the potential required to handle your data cleansing requirements, if they offer the flexibility to upscale/downscale operations, turnaround time offered, and pricing. Hence, you can make informed decisions accordingly.