The Potential Impact of Implementing Data Science Strategies
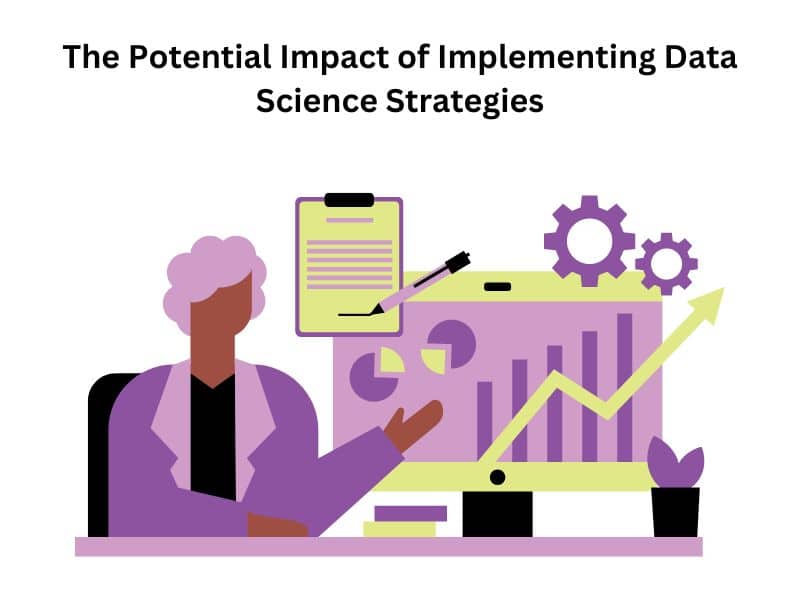
Data science has become increasingly popular in businesses of all sizes for its ability to help optimize performance in the Internet of Things (IoT) ecosystems. By implementing data science strategies, an organization can experience tremendous performance improvements, from automation and efficiency to reduced costs.
When it comes to automated systems like IoT, data science can be used to make them more efficient and reliable. Using predictive analytics, data scientists can analyze the data generated by different sensors and devices in the ecosystem and produce valuable business insights. This helps managers make better decisions that lead to better outcomes for their organization.
Data science also plays an important role in ensuring security and reliability in IoT systems. By monitoring the system over time, data scientists can identify potential threats or vulnerabilities before they arise. With carefully devised algorithms, they can detect suspicious activities within the system and take corrective action quickly if necessary.
In addition, with data science in IoT systems, organizations can save a lot of time and resources by automating tedious processes like maintenance and support tasks. This reduction in manual labor not only reduces costs but also enables employees to focus on tasks that drive real organizational value instead of handling mundane chores all day long.
Finally, there is less complexity when it comes to dealing with large amounts of data from multiple sources. Data scientists use specialized tools that automate the analysis process so human intelligence isn’t required for every task involved with managing an IoT system.
Benefits of Data Science in an IoT Ecosystem
With the rise of the Internet of Things (IoT), data science is becoming increasingly important in optimizing technology performance. Data science can be an incredibly useful tool for developing an efficient and connected IoT ecosystem by allowing for automation, predictive analytics, improved efficiency, enhanced decision making, resource optimization, problem solving capabilities, reduced cost and time savings.
Data Science Automation: Automation is key when it comes to streamlining processes in an IoT ecosystem. By employing data science techniques such as machine learning and AI, computers can be trained to execute tasks traditionally done by a human in a much more efficient manner. This results in greater speed and accuracy, minimized errors and improved customer satisfaction. In addition, automated processes are typically more affordable which leads to further cost savings.
Predictive Analytics: Predictive analytics is another powerful tool enabled by data science that can add value to an IoT ecosystem. It allows for analysis of historical data such as customer behavior or product performance that can be used to predict future outcomes with a high degree of accuracy. This can then be used for proactive decisions about maintenance schedules or inventory management in order to improve operational efficiency and optimize resource utilization.
Improved Efficiency & Data Insights: The use of data science also brings tangible improvements to an IoT ecosystem through enhanced decision making and real time insights into operations. Business intelligence tools powered by data science can provide meaningful visualizations that help identify areas where significant efficiencies can be gained or problems solved quickly. Furthermore, this valuable information can provide actionable insights so that decisions are made based on the best available evidence — leading to improved performance and higher customer satisfaction rates.
How Data Science Enhances Performance in IoT Ecosystems
Data science holds a significant promise when it comes to enhancing performance in IoT ecosystems. By leveraging data science, you can optimize performance in your IoT ecosystem, analyze usage patterns and enhance security & safety. Additionally, you can automate processes and maintenance tasks, streamline networking connections and transmissions, as well as generate insights from large datasets.
Looking at data science through the lens of an IoT ecosystem allows us to gain insights that could help us improve the system’s performance. Data science techniques such as supervised machine learning can be used for predictive analytics which allows us to anticipate any potential risks or issues that could arise in the network. This gives organizations the means to act expeditiously should a problem arise so they can maintain optimal efficiency within their IoT networks. You can also read: Data Science Course in Bangalore
It is also possible to use data science techniques like deep learning for automated anomaly detection which uses techniques such as classification and clustering to identify abnormalities in data patterns. This allows organizations to detect suspicious activity entailing their networked devices and take corrective action promptly. Additionally, data science can be leveraged for anomaly based supervised machine learning algorithms which detect gateways connecting malicious devices or software that are trying to hijack or breach sensitive sensors or connected objects within an IoT ecosystem. Such tactics allow organizations to increase the security of their systems and protect against potential cyber threats arising from unknown sources.
Data science also plays a critical role when it comes to automating processes within an IoT ecosystem by using natural language processing methods such as inference engines or intelligent agents that can interpret user commands, translate them into instructions, and then execute them on their own.
Tools and Techniques Used to Achieve Enhanced Performance with Data Science
Data science is a powerful tool for optimizing performance in IoT ecosystems. Through data exploration, automated algorithms, machine learning models, AI predictive analytics, feature engineering and selection, visualization techniques, and analytical pipelines, data scientists can uncover insights and leverage them to improve the system’s performance.
First of all, data exploration is essential for developing an understanding of the historical patterns that drive the performance of IoT systems. This includes studying the overall landscape of the organization or industry as well as individual components in order to identify relevant relationships that can be used to optimize performance. Additionally, automated algorithms can be used to continuously monitor and analyze these trends in order to identify opportunities for improvement.
Moreover, data scientists often utilize machine learning models to create predictive frameworks that enable them to forecast how a given set of variables will influence future outcomes. These predictions are then used by AI algorithms to automatically adjust parameters in order to maximize efficiency without sacrificing user experience or other important objectives.
In addition to being able to identify and analyze correlations between variables, data science also provides valuable insights into feature engineering and selection. Feature engineering refers to the process of creating new features from existing ones so that they can better describe a system’s behavior. On the other hand, feature selection involves choosing which variables best explain variations in output so that they can be utilized more effectively by AI technologies.
Finally, data visualizations such as charts and graphs offer an intuitive way of displaying complex datasets while generating actionable insights from large amounts of information. It is also through visualizations that data scientists are able to create analytical pipelines which allow them to rapidly test different configurations at scale without sacrificing performance or accuracy when it comes time for deployment in production environments.