Uses Cases for Data Science and Business Intelligence
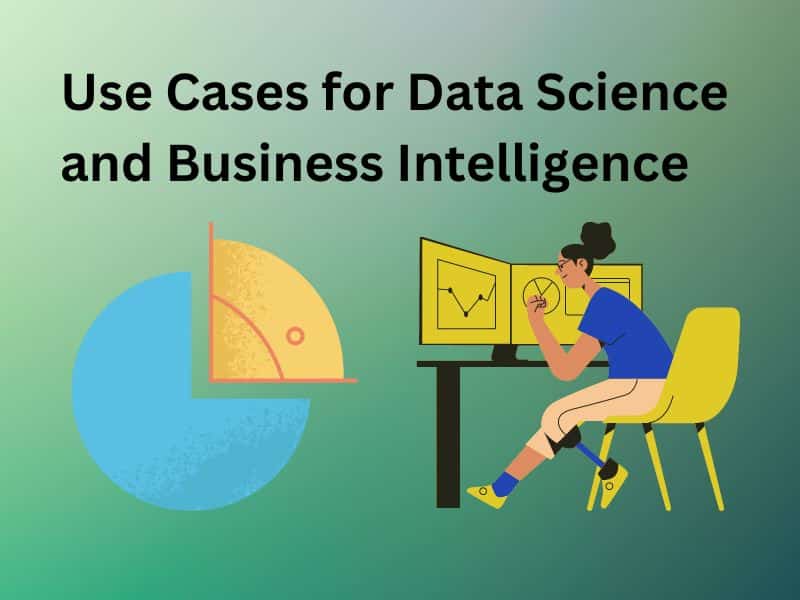
The importance of data science is growing day by day. As businesses look for more ways to gain a competitive advantage and maximize their efficiency, there is an increasing need for data science and business intelligence. But what are the key differences between data science and business intelligence?
Data Science allows businesses to leverage predictive analytics by using big data, machine learning algorithms, statistical models and various other tools to identify patterns in large datasets. Business Intelligence (BI) on the other hand focuses primarily on collecting, analysing and visualizing data to provide greater insight into operations.
Data Science has made it easier for business owners to make decisions based on reliable insights from their data. With the combination of predictive analytics and machine learning algorithms, businesses can more accurately predict how customers will respond to new products or marketing campaigns. This helps companies make well-informed decisions about which strategies are most likely to be successful.
Business Intelligence also offers great value as it provides businesses with a detailed overview of their performance. Companies can track various metrics such as customer engagement or sales growth on an ongoing basis using BI tools. This helps them monitor trends in real time so they can take timely corrective action if needed.
In conclusion, both data science and business intelligence can be used together by businesses to make informed decisions that result in improved efficiency and higher profits. By utilizing predictive analytics, companies can get ahead of their competitors by predicting customer behaviour before they even know it themselves!
Differentiating Factors between Data Science and BI
In today’s data driven world, understanding the difference between data science and business intelligence is essential for business intelligence professionals. Both are powerful tools for managing and analysing data, but there are a few key differences that separate them.
Machine Learning
One of the main differentiating factors between data science and business intelligence is machine learning. Data science involves harnessing the power of machine learning, which is a branch of artificial intelligence (AI). This enables data scientists to create complex algorithms that can analyze huge amounts of data, uncover complex patterns and relationships, and identify predictive trends. Business intelligence doesn’t generally utilize machine learning algorithms since it focuses more on basic analytics such as reporting, dashboarding, and visualizing existing data.
Model Building
Data scientists use statistical techniques to build models, while business intelligence specialists lack this skill set. Data scientists also possess an in-depth knowledge of coding languages such as Python and R to develop these models and run simulations. In contrast, BI tasks usually involve taking an existing report or dashboard and drilling down into the underlying analytics within it—they don’t usually require coding skills.
Data Wrangling
Another key distinction between data science and business intelligence is the process known as “data wrangling.” This is a critical step in making sure that your datasets are accurately prepared for analysis by cleaning out irregularities or incorrect values. Data wrangling requires extensive coding skills, so it’s more common for data scientists than for BI professionals—BI tasks typically involve working with pre-wrangled datasets instead of creating them from scratch.
Pattern Detection
Data science involves detecting patterns within large datasets that are too complicated for traditional methods like spreadsheets to parse through quickly.
Comparing the Benefits & Challenges of Using Data Science & BI
Data Science and Business Intelligence (BI) are two powerful information sources that provide invaluable insights into the operations of an organization. Both approaches offer advantages to a business, and it’s important to understand how they compare and contrast in order to make an informed decision regarding which one is right for your organization’s needs.
When it comes to deriving insights from data, Data Science is designed to take data from across a multitude of sources and analyse it in depth. It’s more suited to discover patterns, trends and correlations that may not be immediately obvious at first glance, by using machine learning algorithms and AI-assisted technology that can process vast amounts of data in a short time. On the other hand, Business Intelligence is focused on collecting and organizing data from siloed sources before aggregating them into reports or dashboards. This approach allows for quick cost analysis as well as scalability and automation of datasets with minimal input from stakeholders.
Data Science offers the advantage of being able to analyse complex datasets with greater insights due to the scale of its operation. However, the accuracy and precision of the resulting outputs are heavily dependent on the quality and format of input data. BI systems rely on structured datasets that have been curated manually, so there’s less reliance on potential errors within raw input datasets during analysis but this can come at the cost of taking away some valuable insight opportunities provided by Data Science.
In conclusion, while both Data Science and Business Intelligence provide powerful information resources for businesses today, they should be evaluated carefully based on their individual capabilities before committing resources towards either implementation path.